Harnessing AI and emerging tech in insurance: A conversation with Sabine VanderLinden
15 minutes
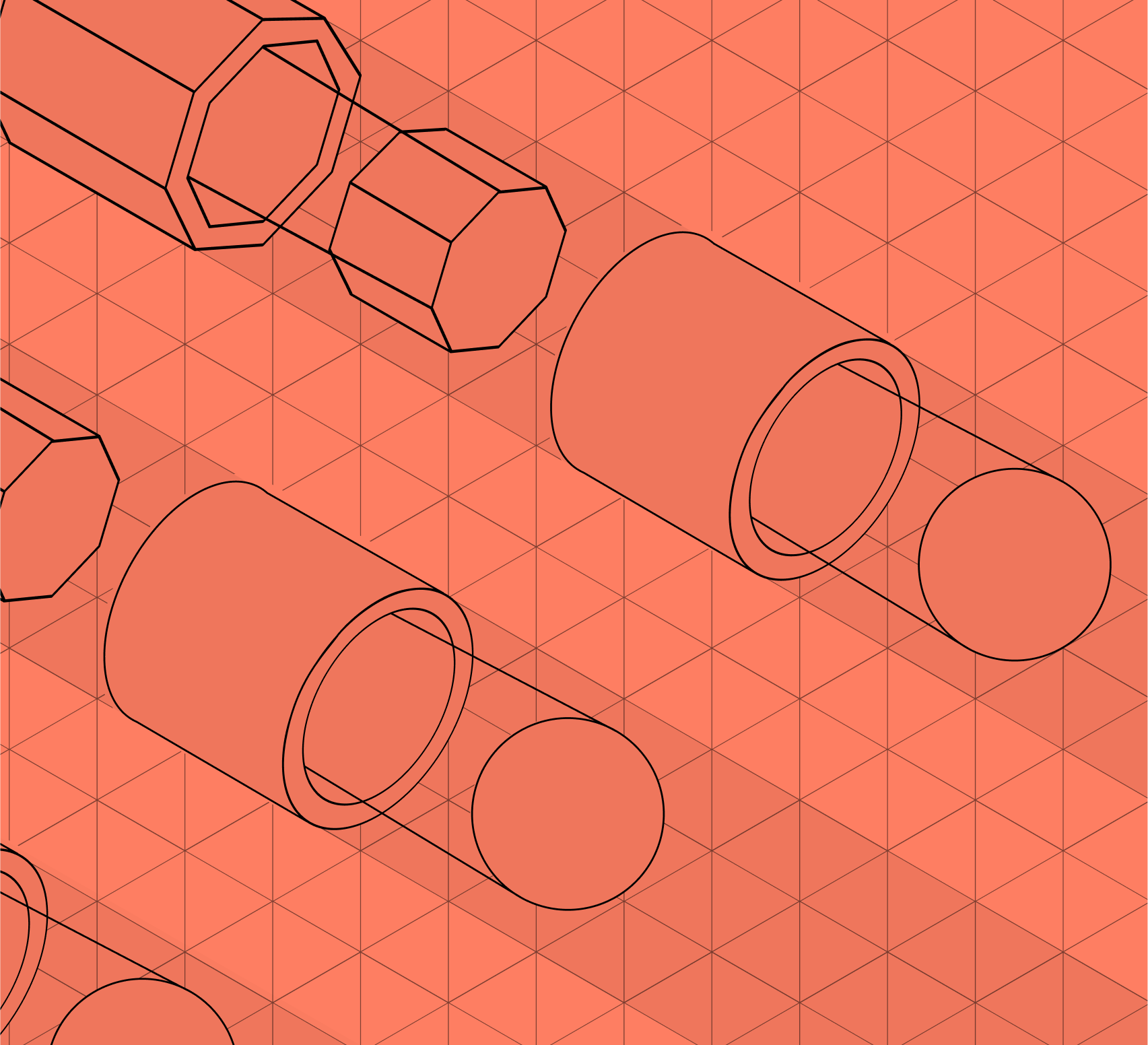
TEC speaks to insurtech and innovation expert Sabine VanderLinden about how emerging technology is helping insurers reimagine their operations and unlock underwriting excellence.
In a world marked by rapid technological advancements, changing customer expectations, and evolving risks, insurers are embracing innovation like never before, but there is still a way to go. To delve into the heart of the emerging insurance revolution, TEC sat down with InsurTech expert Sabine VanderLinden, CEO of Alchemy Crew, about the power and promise of emerging technologies to transform the insurance industry.
From operational efficiency and customer experience to data transparency and profitable decision making, new technologies are already reimagining ways of working for early adopters. The two discuss the benefits of closer collaboration between insurers and insurtech providers, plus the road ahead for wider adoption as these new technologies prove their mettle.
In this episode:
The potential of generative AI in insurance
Collaboration between insurtech and insurers
Data transparency and decision making - including DeFi
Operational efficiency and risk assessment in a changing market
The promise of AI and blockchain in decentralized finance
Never miss an episode
Subscribe on your favourite podcast platform:
"There's a lot of interesting things which need to be done to bring insurance to the 21st century"
Full episode transcript
00:00:00] TEC: Hi, I'm Tom Chamberlain, VP of Customer Consulting at hyperexponential, and your host, otherwise known as TEC. I'm delighted to welcome you to the latest episode of TEC Talks. In this episode, we're talking to Sabine Vander Linden, who is CEO and co-founder at Alchemy Crew. So let's get straight into it. To kick us off, Sabine, we recently did a survey to understand insurer's top priorities and pricing and underwriting are quite naturally, I think, came out at the top, recently overtaking claims and operation transformation as top priorities. So why should insurance companies really care about this and, and, and in particular around sort of innovation, and transformation and pricing and underwriting.
[00:00:51] Sabine VanderLinden: So I believe, Tom, that insurers need to pay more attention to innovation in pricing and underwriting because we are in the world of artificial intelligence, and when you think about AI and partly the past six months where generative AI has become much more accessible, right? For people like you and I, well probably not you but me, to understand how artificial intelligence can be used in business to improve actions.
I don't think insurers have a choice – to remain relevant for their customers, to actually deliver highly customer-centric experiences and support the new risk, emerging risks, which are coming up during the market – they need to really innovate in pricing and underwriting. There's no choice.
And as you know, I coin a statement. Digital or dynamic underwriting, dynamic business models, which requires much more real time engagement, being able to give pricing which is aligned to risk. Think about flooding. You need so much data to actually deal with a flooding risk. It's not static anymore, it's dynamic, and therefore innovation needs to take place around the risk assessment process.
[00:02:05] TEC: That’s all really interesting. And I'm curious now how in Insurtechs are kind of well-placed to help disrupt and transform. 'cause as we know, the insurance industry is lagging a little bit in the, innovation side and seem to be fairly slow to move. And even then, Insurtechs have been around for, for quite some time.
So I, I'm just curious to sort of get your thoughts on, on how they can really help transform why now? Why now is the time really?.
[00:02:30] Sabine VanderLinden: It's an interesting question, Tom, why now is the time? As you said, Insurtech has been going on for quite a few years. I fell into insurance in 1997 and now we are into the Insurtech space and I fell into Insurtech in 2015 actually. And when you look at our Insurtech market, I think it's very important to place market.
Think about FinTech. FinTech is around 690 billion, dollars in investment received by, you know, tech investors. And then in Insurtech represents 67, 68 billion dollars in invested dollars by investors. And so it's around 10%. But as you know, Fintech has been running for much longer than in Insurtech.
And I think we had to go through wave of change. The first one for me was very much around customer experience. You know, a lot of startups focused on driving that customer journey on apps and getting the insurance experience far more digitized.
And then we've had now this period where we have been trying to really understand from an infrastructure viewpoint and looking at much more complex way of doing insurance technology. Looking at the infrastructure and how the components fits together may be more understanding around API and you know, for the past, I guess three, four years we've been around embedded, embedded insurance. Now, I think for me it's around the operating model of insurance and making it more dynamic.
And insurance companies, brokers or insurers, I don't think they can do it too long because of the legacy systems. You know, I talked to a few of these insurers and often there's say Sabine, we have 200 legacy system because of M&A. They have hundreds of legacy system and they have to deal with those when they're actually trying to build those new experiences.
So it's a time for Insurtech because partly the one who have understood how to build an infrastructure led platform, or more dynamic capabilities, as you said, around risk assessment, underwriting, leveraging data, external and internet data, combining it together to provide a better outcome.
I think it's a moment, a very, very timely moment for Iin Insurtech because those who are able to augment the insurance business model and make it more dynamic, help the insurance company be more relevant for their customers, whethe it's a consumer line, you know, or a customer or client, like a commercial line client, and I think commercial lines, which is much more complex, takes a much more time to actually understand the capabilities that are required to make that transformation.
Those companies, I think they're not all Insurtech. Right. And I'm sure you would agree with with me, where you have a lot of tech relevant for insurance like cyber for instance. And I think this is a really relevant moment because that's the only way we can differentiate partly in a market where, you know, capital is scarce and customer are going to fund the best deals which are around.
[00:05:37] TEC: Yeah absolutely. And, and we've seen evidence of this, right? So Convex who are I guess, a startup in the insurance world been going for just about four years now. They very much started as a kind of tech first company. So, and, and obviously being, being sort of in a nice position not to have to worry about the legacy side, which you just mentioned, and been able to create everything from scratch.
But they, they really have created a futuristic business model because they use different tech stacks and they very much embrace that side of things, but they've been able to move quite quickly and grow incredibly rapidly and successfully. It's a real big lift, I guess for some of the more embedded insurers who, who do have all of this huge legacy side and it is a bit of a burden.
And your point, I think you're right. I think ins, Insurtechs and technology companies, data companies that they, they could all help with this, but I still feel it's going, it's still going slowly. You know, again, you, you said you were getting into the Insurtech world sort of 2015 and I think actually we're working at a similar time on companies with like flock.
We're doing exactly what you talked about, right? The, the distribution side and being able to make insurance much more accessible to, to consumers. And now, as you said, we're seeing this sort of shift more towards this operational efficiencies and, and, and helping out and, and do, do you think there's been a sort of real big leak forward then in, in that sort of capability side of things?
And maybe that's what was holding it back before, you know, it was maybe more experimental bits and, and helping out, but now maybe it's a bit more tangible and you can really see the effects and the benefits it's having. have you, have you seen that sort of big leap in the last few years?
[00:07:16] Sabine VanderLinden: So I've seen amongst insurers and I think, you know, let's assume there is around 5,000 main insurance company across the globe. I would say innovations still remain done by the early adopters. Think about crossing the chasm, you know, early adopter, fast followers, laggards.
I think the adoption is still within the early adopter. And then you have the fast follower who actually want to play catch up, which are going to implement things that they have seen iis working across other organization and so.
I do feel that many insurers are trying to do it and they are learning to collaborate more effectively with startups. But you know, it's also about culture, internal culture of the business. And this takes forever to change. You know, I remember when I was doing a lot of social economics and looking at social science, which now I think is moving to prom science, right? What I, I used to see is that it's all about the culture, and the culture takes time to change.
It takes an average seven years to change a culture actually on average. So I think many insurers want to do it, but don't always know how to do it. And when they do it and fail at it, they actually stop the initiatives while actually they should stick to it. Those have seen succeeding, have actually in some way industrialized and democratized specific techniques.
And this is the type of things we've been doing with some of them around, you know, creating adoption framework, commercializations framework to enable them to adopt technology, and started within the operation. But I don't think it's a masses, actually, Tom, I think it still remains the few because of the risk version of the industry.
You know, the fear of failure and so when something doesn't work they park it and then you don't know when they are going to go back at it. Think about big tech. If they fail, they go back, right? Because for them it's a lesson learned and I think the industry needs to be better at appreciating lessons learned – let's not call them failures – lessons learned, so that they can actually build much more resilience and responsive business model.
And I think that's what we are moving towards, right? We are living in a world where sustainability is top of mind. To do sustainability right you need to understand your tech stack, but you need to make sure it's green, but also you need to understand what it means for your customer. Moving from fossil fuel, for instance, to renewable. Or what it is to manage environmental risk like flooded wildfire, earthquake, you know, all those environmental stuff that a lot of insurers are looking at, particularly in the Lloyd's market.
And that requires new technology. It requires new data sets, right? Adding to your existing data set, it requires for your underwriting, your assessment to be much more real time and dynamic. So, all these algorithmic stuff that we have heard from Key, Convex, you know, a lot of young players, you know, the one with the greenfield tech environment that they have been able to implement to serve their customer better.
And then looking at what the business model could look like using technology, for example, to do circular. To do circular, you need technology. You need to understand IoT sensors, how you take the data out of the sensors. How you use digital twins to actually understand where a property may be at risk of, physical event.
All these come together and requires more technology and more detailed assessment, deep assessment to predict and prevent, right, to actually provide the customer with better insight to actually take the right decisions. So few, I would say, but I think more and more because. Relevancy becomes much more important and ensures if they want to survive and still remain, they would need to start using better tech to serve their customers.
[00:11:22] TEC: Absolutely. something you said they really resonated. You know, you, you backed away from the word failure. But actually I think, I think it is that it's, it's having the ability to fail fast, as you said. The, the big tech companies, that's exactly what they do. They, they work agile, they fail fast and, and iterate and carry on.
Insurance companies tend to do a huge waterfall project, which is gonna take five years, and then a surprise when it doesn't happen. And then everyone has the fatigue of like, oh, we, we, we just can't do that again. And it's, it's this combination of trying to do it themselves. You know, they're, they're not big tech companies, you know, they're not technology companies.
Insurers incredibly good at doing insurance. That's their reason for being, they, they're there to understand risk. They're not necessarily there to create technology. And I think when you try and bring a couple of those things in together, it can be sometimes a bit of a mess. And don't get me wrong, some companies have done it and done it extremely well, but is very, very hard to get right.
And this, this fatigue, again, this, this how long it takes to do transformation is such a huge barrier sometimes. And then you have that added bit, which you said afterwards, which is the culture change. You know, it's, it's all very well embedding this great technology, but. If you don't have the culture, then to go and use it to the right way.
You know, you go and be data-driven underwriters left to their own devices Will, will continue to do things the way they've always done. And it's, it's served them well in the past, but as you said, that they're gonna get left behind and they need to have that mindset change as well as everyone else in the company to go and adopt this technology and, and do some new things.
Those ones, those few that you mentioned, they're, they're gonna be the ones who are leading the market in the coming years, and as you said, people will be left behind. And so have you, have you got any sort of. Tips and tricks, I guess for, for insurers. Sort of listen to this and, and, and about how to adopt, like things like, Insurtech, because it's not always an easy thing for a company to do.
As you said, they're quite risk averse still, and the idea of embedding a small kind of startupy scale-upy type of company can sometimes be a little bit daunting and goes completely against their risk version. What would you be saying to those companies?
[00:13:30] Sabine VanderLinden: I guess first I would try to explain to them the dichotomy or the relevance of looking at insurance and Insurtechs as two groups, which can overlap. So think about the insurer himself, or the company, which is the insurance organization. The corporates can actually provide so many resources for an Insurtech.
They may have the willingness to partner and invest. They will have, you know, the global infrastructure and the global reach as well. They have often brand reputation. Think about Lloyds of London, it has been in market for 335 years. Think about brands such as Generali is 110 years old. Zurich is 150 years old.
So those companies have over years built brand respect and a reputation, which is, you know, which is incredible. And so those are important because they're there 4 million of customers. Often, whether they are corporate customers or individual customers. When you think about PNC, they also have core capabilities and they have been building those core capabilities.
I know we talk often about legacy, but think about core capabilities beyond legacy. They have underwriting teams, they have actuary teams, they have claims team, they have experts. And for example, when I think about Travelers, Travelers in the US as one of the few Claim Excellence Academy, right? They even build academies around their expertise.
And then they also have distribution or can access distribution through brokers. So if you start now looking at it as a digital ecosystem, you can link the insurer, the broker, and the re insurer. And this become as one an interesting digital ecosystem to to touch for the Insurtech. Now for me, the Insurtech and the Insurtech founders, they want to solve the problem.
They want to change the world, right? So when they went to build an Insurtech there. their goal was, was to develop a disruptive ideas. And I know in insurers, in insurance, we don't like the word disruptive. Let's say they want to reinvent our industry and build capabilities. Transform, right? Reinvent. Disruptive is always a bit tricky. However, they always use the latest technology and you know, when I started in Insurtech in 2015, I saw Insurtech using own stuff, right? But very quickly they realized the only way they can be called Insurtech was by using the emerging tech, you know, the, the tech which can transform the industry, the big data, the ai, the blockchain, you know, and the cloud.
All those stuff which allows to, to build a really personalized and rich experiences for the customer. Then they also have their own network. Founders have connection with other founders and you bounce idea all the time as to where the market is going. And then I think which is important is this entrepreneurial approach where you are able to work with very resources whilst corporates, you know, there's a lot of waste, I'm sorry, corporates, I love you, but there is a lot of waste and therefore, because there is a lot of waste, it can take a long time to get things done.
And the Insurtech always look for new business model, tend to look for new business model. That's the goal. They don't always succeed, right? But that's the goal of an Insurtech. So when you have the, the tool overlapping, you can have a really dynamic reaction. And so for successful implementation, you need to have a process.
You need to have a framework. And for me, the frameworks start with strategic intent alignment between the two. But at least for problem, we want to source. So let's say we want to solve for assessment and we want to make it more dynamic. Then we start looking for companies, whether those are data geospatial or we look at underwriting capabilities, which are a bit more dynamic, right?
Like what you are doing, hyperexponential. Then we look at the cohort, right? We don't look at one, we look and compare the cohort to see what could be good capabilities to solve the problem the insurer has in place. And then it's about building that evaluation. Discovery and adoption plan, and so that require very clear understanding of, you know, do we have a customer?
Is the customer going to make money? And what are the capabilities which can solve for the problem, which not always, all the capabilities offered by the in Insurtech. And I also said to Insurtech, it's fine for you to have all these 20 key capabilities, but look at me. I'm insurance company A, B, C, I only need three of your 15 capabilities.
So you need to put it in my, in my terms, in my ways so that I can actually talk to my underwriting team, actuary team. If we are looking at an internal user and then them looking at their own customer to see, okay, these can enable me to manage my portfolio better, these can enable me to find capital easier, these can enable me to serve that customer faster and therefore potentially take customers from are own competitors.
And so I would say it's about problem statement. Strategy, then it's about alignment of capabilities. You know, can we work together? Do we love each other, the culture. And then it'll be about the framework. You know, the, the how we are going to talk, start working together. And, you know, often said to insurers, you need to have, dichotomy or a double process.
You have the one use with, you know, the like of the big, the big guys, right? The IBM, the AWS, the, the companies were being. Proving themself for many years. But then for tech startups, you need another process. You need another evaluation process, which is lean and faster, which is not going to take six months because startups have very limited resources. It has to be done within three to four months, full stop, and then everybody's happy if it goes for yes or no, but at least no one is wasting resources.
And so it's. About implementing those capabilities internally, which is often the hardest because you have to do a lot, a lot of convincing, and it's never done the first time. Right. It's when you interact, even I, when I build the acceleration program, first year was very different for second years, which was very different third year because you tweak and refine your, your approach based on the startup and the corporate you're working with.
[00:19:40] TEC: I couldn't agree more. The, the alignment piece is, is really critical. It's, it's interesting that you say you need to have this kind of double approach, you know, one for the big, the big corporate tech companies like the IBMs and then something different for, for the Insurtechs. And, you, you have to be, it's quite interesting.
You have to be a little bit more used to, to going a bit more agile, going a little bit quicker with the Insurtechs because they wanna run really, really fast at this. And I, I do remember talking to a few of the cohorts actually, back in the start of startup bootcamp days and, and actually. Helping this on the other side as well, because in Insurtechs, they're, they're expecting things to happen now, now, now, now.
And actually when you send an email to a big corporate, you could probably expect to wait a few days, if not a week for a reply. It's not because they're ignoring you, it's just because that's how long things take. And having that alignment on both sides is, is, is really important because otherwise one of the other signs is gonna be frustrated.
And Insurtechs in particular, get constantly annoyed and why is it going so slowly? Why is this not going? And, and forgetting that sometimes, no, it has to go to this person, this person, this person, before any kind of further decision can be made. And it's, it is a bit tricky and I think there's, that also probably leads onto, again, corporates - They need to have a different approach to doing this. You know, you, you can't, as you said, you can't have the same approach for a massive tech companies. You can, for these small ones, things have gotta move quickly. Otherwise, again, you're just wasting time. You'll be left behind.
You know, a lot of the transformation the Insurtechs are talking about can be implemented in weeks if not months rather than an IBM level transformation and will take years, right? That's because it's gonna be inevitably a huge project. So the ability to move quickly and want to move quickly is, is key. And again, I think there are some, some insurance companies out there who are, who are much better than that, than others.
But it's, it's still interesting to see there is still this. This barrier sometimes to, to getting these things over the line. But I really liked how you framed it. It's, it's the problem statement, right? It's understanding what, what is it, what is the problem needed to be solved, you know, is it, is it underwriters are spending too much time doing data entry?
You know, is it I can't have, don't have access to my data in real time, whatever those might be. And then picking and choosing carefully. Which of these tech companies, which of these Insurtechs are gonna most clearly align to that and be able to deliver what you want. Because that, that, that's what it is at the end of the day.
Right. It's, it's, it's solving a pain point and, and providing a use case for whatever it is you're trying to solve.
[00:22:00] Sabine VanderLinden: I wanted to actually share with you and just give you a use case. I was solving recently for geospatial, you know, we are learning about geospatial and the problem was solving for was between flooding and wildfire. And through my learning, I realized that actually you cannot solve wildfire and flooding in the same way because both are dynamic risk, but flooding is a much more dynamic risk than wildfire and you need much more data for flooding.
And so therefore the capabilities you identify for one would be very different for the other 'cause you are looking for, you know, how close you are from the physical risk. And so the population, I would say the cohort of venture would be very different from one to the other. Very, very different actually from one to the other.
[00:22:45] TEC: It's interesting, I'm, again, I'm there, there are so many use cases out there at the moment, and, and there's so many of these little spots there. It's it's also a prioritization thing, right? For companies where, what, what's, what's gonna give you the most margin? What's gonna help you? Right now, and I think a lot of companies have been through big process transformation and, and sort of trying to streamline that.
And, and now it's what, where, where can you leverage the best data that the better short text, if it's, if it's geospatial, if it's understanding the data or, again, a little more on the operation side and into things like you mentioned earlier. Right? So, Machine learning and ai. These are, these are big things at the moment and I know we've been talking about them for, for some years.
And have you seen any sort of good recent examples of from the Insurtech world about machine learning and AI relating to this big corporate specialty insurance space?
[00:23:35] Sabine VanderLinden: I have, and I think for me, the space, which is exciting as you, as you probably know, is, the underwriting and risk assessment space, because there are now a number of players who have been perfectioning their proposition around big underwriting, dynamic underwriting or augmented underwriting, because now we also have the European Commission Artificial Intelligence directive.
And so it's not just doing AI. You need to have AI, which is explainable, which is transparent, which is responsible, which is trustworthy, and you know all those keywords. And then you also have a human in the loop to actually make sure that we can create some guardrails to make sure we are serving the customer and we are not pushing things which are irrelevant.
However, you would not doubt it, where I'm getting a lot of question at the moment is around generative AI and the usage of it, and I think it's important for people to realize and understand that generative AI is beyond just chat GPT or GPT 4. There is two other types of I would say generative AI.
We need to actually be really, we, we need to understand we have Bard, which is cool. I think it's a bidirectional encoder representation from Transformers, right? Very long mouthy stuff. And then you also have the Transformer Excel. And the difference is the Chat GPT is prompt based. So you know, we put a prompt and you get an answer, but the Transformer Excel is long text.
So why I'm mentioning that is because at the moment I think you have a lot of experimentation in legal tech using generative AI to actually start looking at comparing contracts or even rewriting contracts, making simpler. You also have a virtual assistant, right, which can now be much more human. Using the avatar and I'm getting my avatar being built. Actually, Tom, I'm very excited.
But also you have the chatbot. So think about basic chatbot, right? We move from FAQs to chatbot, and now you have the generative AI being able to do the next version of chatbot again, which are far more human. I mean human in the style of the writing. And I'm not saying the AI is human, I'm saying it's much more human in the style.
However, it's, we are still early stage and so there's so much more to be done. But all these can be used in claims underwriting, fraud detection. So think about the negative we heard about fraud and cyber. That Chat GPT can create code, which actually can create malware. But think about the reverse. Chat GPT also can identify code that can prevent fraud and can actually identify the malware which was created.
So you know, there is an even ended positive negative around both side, but the technology is there now to a point where it'll be transformational. Transformational. That's work game changing. We are at that stage, I think now.
[00:26:26] TEC:I agree. Actually, I was, I was chatting to someone the other day about, chat GPT and, and trying to sort of understand that there is still, there is still some inherent, I don't wanna say dangers, but that there's, you've got to be very careful with, with how it's. Used right, you, you mentioned it earlier, chat, GPT, Bard, whatever choose your generative AI of choice, that there is a low barrier to entry now, to, to use these things.
And before, if you wanted to create a machine learning, algorithm, you know, th this requires a fair amount of skill, certainly beyond sort of my technical capabilities. Some of my team very, very good at that. But there is, A lot of design and intelligence put into creating the, the algorithm, the machine, for doing that particular purpose.
With, with generative ai, now it's very easy just to jump in and start asking it questions and, you know, you, you, you and I will have grown up with sort of in, in internet sort of, Strategies where you don't just use Google as a yes no answer, right? You would never put anything into Google and say, what is this?
And take the first answer as completely…
[00:27:28] Sabine VanderLinden: You would scroll? Yeah.
[00:27:29] TEC: Right? And, but the, but with Chat GPT, you can ask it something and it will give you what seems like an incredibly credible answer unless you are. Potentially an expert in that sort of field. And there is a bit of a danger here that it's just used as that sort of black box thing and, and it can regurgitate a whole lot of bias, which the, which the data, it's, it's based on.
And you need to apply some level of sort of intelligence and expertise over the top of it to make sure that you are, you are not just doing the Google. And yes, that's my first answer. but I don't think there's any way around it. Right. It's, as you said, the, the, the cat's outta the bag. It's here now.
And we've got this going forward.
[00:28:07] Sabine VanderLinden: And we need to continue with it, right? We can't say, let's stop because you are going to have winner and you are going to have loser because people, I mean, Google, Amazon, you know, or Microsoft with. Well, 11 billion into Open AI, they're not going to stop. And so I think it's very important for everybody to understand it's here to stay, it's going to be there.
But as you said, and I, I play with it, you know, for research, I would say, but I know it's, it's just to draft. It's just helping me think through. Okay, instead of taking three hours to write an article, now maybe I can write it in two hours because it gives me my first outline and then then based on that, I may like it, I may dislike it, but then I can go and do my research and check every single facts.
So as you said, you need to be an expert. You need to actually identify the crap. Which come out of it. And if, I'm sure if you put your name Tom Chamberlain and I put mines Vander, and I look at it, it's like probably 80% right? 20% crap. And so you just need to be mindful that expertise and experience actually probably will be required with this type of capability.
[00:29:12] TEC: Absolutely. It's how you use it. There’ll be very powerful use cases that you said it will help you suddenly take a job, which is gonna take you a day down to what you just said, 12 hours down to a couple of hours. But I've seen people using it as part of their teamwork and rather than, I did it when I was created spreadsheet many years ago and, and having to like look up how to do suddenly investive embedded if statements and, match index lookups and other things, which like, I don't quite remember the sequence of this, and you can go Google it to check and it'll take you a few hours, but you'll get it done.
Chat GPT can give you the answer just like that. And, and it just accelerates your, your ability to do the work. I really like the phrase I came across. I've been telling everyone this.
It's, you don't need to be worried about being replaced by, by AI or, or generative ai, but you may well be replaced by someone who knows how to use it really well.
[00:30:03] Sabine VanderLinden: Correct. You have something that is probably one I would reuse, Tom. This is absolutely true because what you're saying as well is the jobs of the future are changing. And as you know, MIT is making massive investment in prompt engineering and prompt science, and I'm sure other big university are doing the same.
So now when you think about artificial intelligence or our jobs, we have to become good prompt writers, and that is what I realize. Prompt engineering is about learning to write for ai, and it's a skill.
[00:30:38] TEC: Absolutely. So, do you see this, you know, with all the innovations you're seeing across the market, is it, is it generative ai? Is it machine learning? Is, is this gonna be the sort of next massive leap forward or are there, are there other things kind of. On the fringe of coming, which you've seen, which could, could, could be equally transformative.
[00:30:55] Sabine VanderLinden: So for me, you have the underlying technology and I think it still remain in the sphere of data and you know how use data. So I think we are going to see more data orchestrators because we have so many data providers, think about geospatial. There are hundreds of them, and so at some point, and all of them have different layers of it. No one is perfect.
So when I took to the geospatial company, they said, you know, they have built coalitions, you know, associations so that they can actually augment one another, trying to do, do everything themselves, which I think is superb. Because even from an insurance viewpoint, you need an orchestrator.
You cannot just take one generative AI company's data to do the job you need multiple. And actually it's expensive. So then I think it's going to be then about the business model and the pricing model. So everybody using the these, geospatial data, then it becomes slower. So then I think there is something around data, big data, and the future of data.
Which means geospatial, but digital twins, you know, getting a twin of you, my avatar, but also getting a twin of a building of a physical asset and still you and I sitting in our offices to understand where the risk lie rather than having to travel to this location. So going into a bit more sustainability thinking as well.
Then you definitely have the AI, the machine learning, the different models. You know, the large language model is just one of the many. It's a form of machine learning. Actually it's one of them, but I mean, I can't even remember all the models, but you know, random forest and also and and so on and so forth.
You have so many different types, and it's why I was mentioning Bard, Chat, or GPT 4 and Transformer Excel because Transformer Excel allows you to do long text. And so Bard do comparison and Chat is a prompt. So based on the problem you are solving for, you need to use a different type of model. Not all are suitable for everything.
And so then you have, I think the DeFi, decentralized finance. I think when you start looking then at the Metaverse and web3, which is coming, and I know it's not linked, Web3 and the Metaverse. But when you start looking at the immersive environment and then you and I doing our work with our digital twin, with our VR glasses into those digital immersive environment where our brain is able to process more information in the way we want to learn.
So I don't think it's one technology, I think is a multitude. Of them and why DeFi and blockchain? You know, we are, and don't take me wrong, I love Nigel Walsh and I really appreciate and respect the companies he is working for, however, Google, Amazon, and the big tech owns the world. In some ways, DeFi allows us, you and I to become potentially owners of our data and the stuff we do.
So I think in combination of those can actually lead to interesting outcomes. I don't know what those outcomes are. I will just keep it simple to insurance. Insurance business model with this can be super dynamic and it'll have to be taken in piecemeal. We won't be able to do everything in one go.
[00:34:09] TEC: It is genuinely an exciting time. I don't think in my sort of career lifetime I've seen anything quite as big coming or. Right here now, as, as, as this, potentially could be. It's, it's gonna be groundbreaking. And those companies who find the really good use cases for it, they're gonna be the ones, as you said before, they're gonna be the winners of this game and they're gonna leave everyone else behind.
Interesting, interesting stuff. So, Sabina, I've got one last question, which, which I'm asking to everyone, and maybe if we take sort of generative AI outta the, equation. What do you think insurers, reinsurers, MGAs need to be doing now? To prepare for what? Well, I hope there isn't gonna be a soft market, but inevitability reason that there may well be, but what, what?
What should they be doing to prepare for a potential soft market?
[00:34:53] Sabine VanderLinden: So, at the beginning of the year, I was talking to the deputy editor of the FT on a podcast and she was saying to me, you know, Sabine - I was doing a a trend podcast with her - So, you know, Sabine, the biggest things which are going to affect 2023 will be operational efficiency. Everyone will be looking at operational efficiencies,
So, I think the technology, which is available right now, and I've heard it even this week, again, can actually take percentage points out of the business from an operational efficiency viewpoint, not two, three, 5%, it goes into 20%, which I thought was fascinating. When you start implementing with the technology, we talked about taking out the generative ai, partly artificial intelligence, think about cyber fraud, claims underwriting, so on and so forth.
I think it'll allow the market to look at much more diversified portfolio, a better understand what is making profit, what is making a loss, coming out of specific classes and actually entering new classes. Because of the transparency of data, we can make better decisions. And I think the part around decision making will improve more and more and we'll be able to make it much faster as well.
And then it's all about, I think, the customer. The consumer and the client being able to enable them to understand better why they are being insured against which risk and why the premium is X for that risk. Because then you can actually even provide a dashboard for them to actually understand. The risk profile.
I've seen that in supply chain management. I've seen it in physical risk evaluation where you know you have a flood, you have a hurricane, and you can see around the supply chain or where the properties are, what is going to be affected and what is going to be the impact on the revenue of that, of those companies.
So maybe we are going to see more innovation around business interruption insurance. You know, this type of, or cloud outage, you know, this type of insurance which are paying out when something happened, like an event where, you know, parametric is really placed for that. And as we get more data, we may actually see more parametric propositions as well.
So I think for me that is what insurance reinsurance, MGA need to think about. At the same time, there is a risk, right? There are buyers and we still need to remember there's a regulator and we need to to serve the customer. And apply the right conduct. We need to, to make sure we look at data. I mentioned the ECI directive, so this is going to be top of mind, beyond GDPR, you know, data issues, privacy, security, all this will have, will heightened over the years, not just next year, but over the years.
And then, you know, I wish everybody could be a convex or key and come up with a greenfield business model. But we need to deal with legacy as well. And so how can we do that better? Do we need to build layers, more APIs? You know, there's a lot of interesting things which need to be done to bring insurance to the 21st century.
[00:37:53] TEC: Absolutely. Yeah, there's, it's what we were talking about, right? It's, you need to start making these changes now because they're the ones who have already started off in that journey. There's ones who are well, well into it, and you definitely do not wanna be left behind in a, in a soft market, let alone a, let alone a hard one.
And I really do like, the transparency thing is, is really key. Being able to explain how you've made these data-driven decisions and, and for the customer to really understand what is being assured, what's not. And all of that is super important. So yeah, that's, that's really great. So yeah, that draws us to a close.
Thank you so much for joining me, Sabine. And I'm very excited to, go and find out how you get your own avatar created. I think that's gonna be on my weekend list.
[00:38:33] Sabine VanderLinden: Send me an email and I can share the secret.
[00:38:35] TEC: Oh, awesome. Awesome. Okay. Thank you very much.
[00:38:39] Sabine VanderLinden: My pleasure. Thank you, Tom.
[00:38:42] TEC: That concludes another episode of TEC Talks. If you enjoyed today's show, wanna find out more about the topics discussed, head over to hyperexponential.com to gain access to a range of resources related to this episode. The link is in the description, and of course, wherever you're listening to this podcast, make sure you like, subscribe, and leave us a comment or review.
Thanks so much for joining us and see you next time. Bye.