Written by Risa Ryan
The transformative power of AI in Underwriting: Exploring practical applications
5 minutes
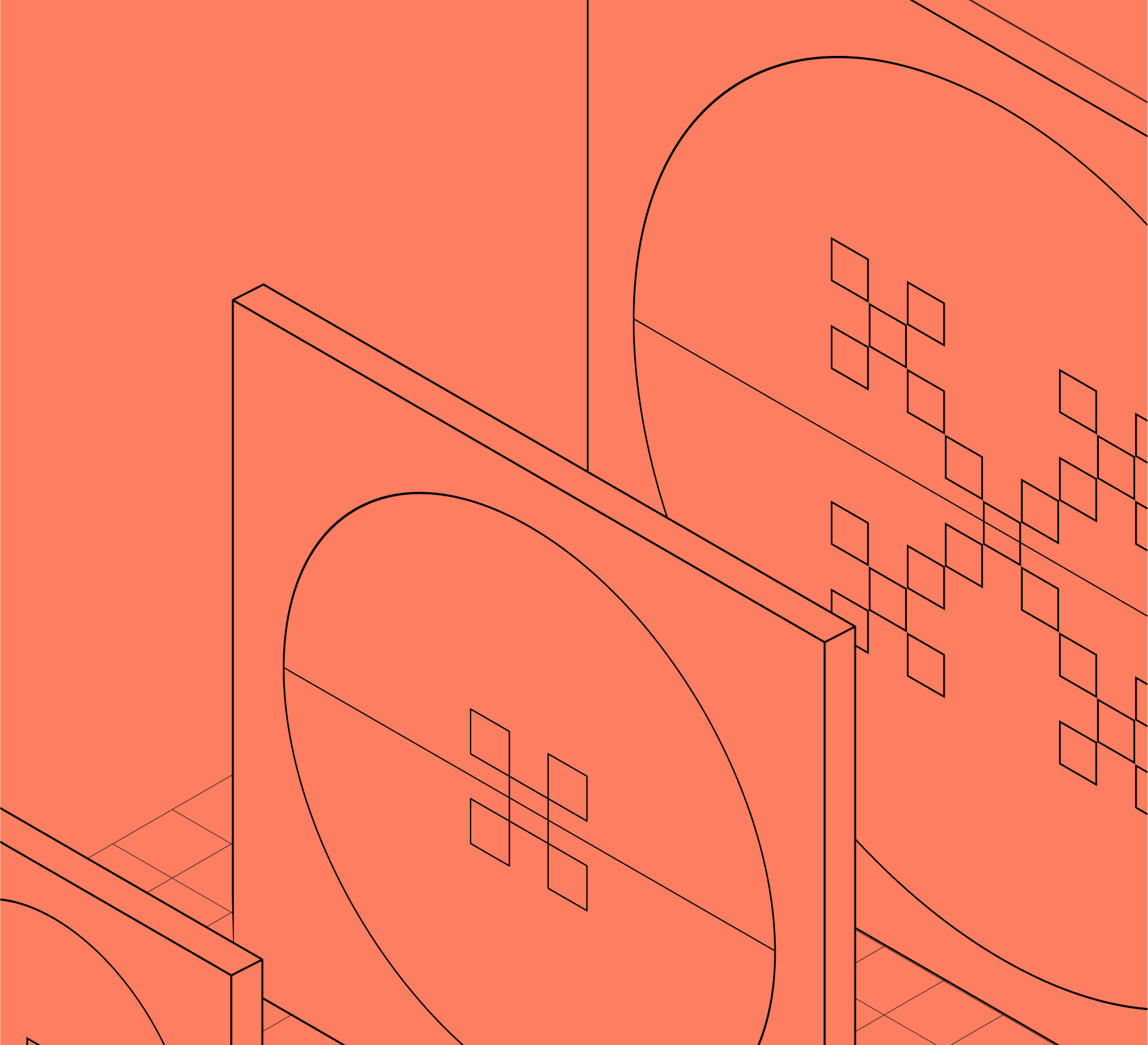
With experience both as an actuary and underwriter, Risa Ryan has spent more than 25 years in the insurance industry. In this blog, she shares her thoughts on how AI and ML will address key challenges for underwriters.
The insurance industry stands at a pivotal juncture, with Artificial Intelligence and Machine Learning poised to resolve several longstanding challenges faced by underwriters. Tackling these challenges effectively will ultimately enable insurers to make better risk assessments and data-backed pricing decisions.
This article will delve into details of how machine learning and AI can provide robust solutions to some of the industry’s most pressing challenges, and aims to explain the practical applications of how and where this exciting new tech will sit in the underwriting workflow.
Challenges in Underwriting
The insurance industry has often fallen behind other financial sectors in embracing and adopting new technologies, leading to inefficiencies and missed opportunities, including:
Manual data entry: Rekeying in insurance is commonplace, with our 2024 State of Pricing Report finding that underwriters spend on average 3 hours each day manually entering data. This process is prone to inaccuracies with resulting errors cascading into pricing discrepancies and affecting the insurer’s profitability and risk management.
Fragmented data sources: Underwriters often need to access multiple sources to gather the necessary information for proper risk assessment. This fragmentation can lead to inefficiencies and potential data gaps, restricting underwriter's ability to make informed pricing decisions.
Inconsistent risk assessment: The effectiveness of risk assessment varies significantly among underwriters based on their individual willingness to explore multiple data sources, their depth of research and underlying inquisitiveness. This variance introduces noise and bias into the risk assessment and pricing process.
Unreliable claims/underwriting feedback loop: The feedback loop between claims and underwriting is often inconsistent, limiting the team’s ability to learn from past claims and adjust underwriting practices accordingly.
AI-Driven Solutions
AI technology offers several innovative solutions to these challenges, particularly through the analysis of unstructured data.
Enhance data entry with Natural Language Processing (NLP): NLP can read unstructured data to prepopulate submission information, minimizing errors and inaccuracies in data entry. This automation not only reduces the risk of incorrect pricing but also frees up internal resource to focus on more impactful tasks, thereby improving overall efficiency.
Streamline data access with image recognition: AI can significantly reduce the need for underwriters to access multiple data sources by leveraging automatic image recognition. For instance, AI-driven predictive models can analyze images to determine the number of stories in a commercial building, roof type, roof quality (e.g., standing water presence), and building age. This reduces the reliance on manual data gathering and enhances the accuracy of the information used in risk assessments.
Ensure consistent risk assessment: AI brings a standardized approach to risk assessment, ensuring consistency across the underwriting process. By employing a uniform AI-driven methodology, disparities caused by individual underwriter's diligence or interest levels are eliminated.
Improve feedback loops with claims analysis: AI can analyze claims data to identify causes of loss, attorney involvement, and claimant sentiment. This information, when fed back to underwriters, enables more informed decision-making: by understanding past claims better, underwriters can adjust their practices to mitigate future risks more effectively.
Fraud Detection: AI can analyze unstructured data from social media to automatically detect fraudulent claims. For instance, a workers' compensation claimant who posts photos of a weekend ski trip can be identified easily. High-resolution drone photography also helps in quickly identifying property damage after storms or catastrophic events, aiding in accurate and timely claims processing.
Sentiment Analysis: Understanding the sentiment of claimants through their communication with adjusters can help identify customer service issues and the potential influence of sentiment on claim outcomes. This analysis can lead to proactive measures to improve customer satisfaction and claim resolution.
Unlocking AI use cases and their benefits
In the short term, AI enhances efficiency by streamlining processes, helping to reduce expense ratios. In the long term, insurers that adopt AI technologies will likely achieve lower combined ratios compared to their counterparts, providing a critical competitive edge in the market. By embracing advanced technologies, insurers can achieve greater efficiency, accuracy, and profitability, thereby positioning themselves for long-term success.
Want to learn more about hyperexponential’s view of AI, machine learning and the readiness of the market to embrace new technologies? See what our Founder and CEO, Amrit Santhirasenan, had to say in his recent blog post here - Bridging the expectation chasm: Insurance and the impact of AI